https://doi.org/10.71352/ac.56.069
Theoretical background of numerical-based input
data extension and reliability analysis for machine learning
Abstract. Nowadays, Artificial Intelligence (AI) and Machine Learning (ML) models are becoming increasingly widespread in practice and every area. The central issue of AI and ML is to create the best model based on the available input data. Building and choosing the best model are often not trivial tasks. These models usually work well if the quantity and the quality of the training datasets are appropriate. Thus, the process of the model building and the raw data itself interact: the characteristics of the data determine the possibilities of the model building. However, data are not always available in large volume. Although smartening industrial, or any other environments up has been going on in recent years, it is still a problem when the data are very 'homogeneous', i.e., there is a relatively large amount of data, but much of it is irrelevant from the point of view of AI or ML modelling. Thus, false models can be built that do not work properly, especially if there are changes in the characteristics of the data. In this paper, the theoretical background of two new, self-developed methods are presented which are able to systematically increase the volume of the input data and examine the reliability and the stability of the applied analysis method. These two methods are able to work together in a framework and can extend the use and the applicability of AI models with new, systematically generated datasets.
Full text PDF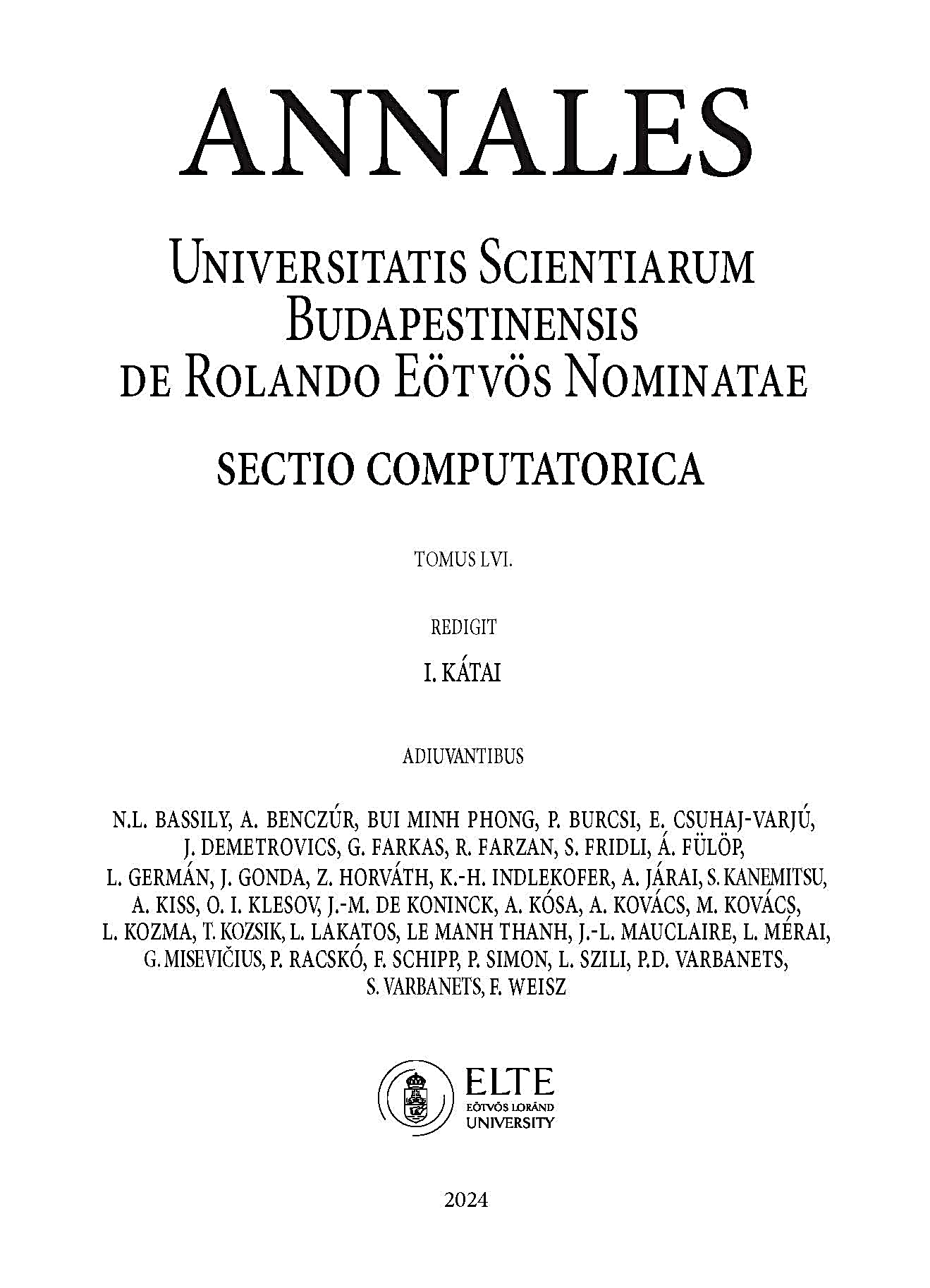