https://doi.org/10.71352/ac.55.097
\(q\)-deformed and \(\lambda\)-parametrized hyperbolic tangent function based Banach space valued multivariate multi layer neural network approximations
Abstract. Here we study the multivariate quantitative approximation of Banach space valued continuous multivariate functions on a box or \(\mathbb{R}^{N}\), \(N\in \mathbb{N}\), by the multivariate normalized, quasi-interpolation, Kantorovich type and quadrature type neural network operators. We investigate also the case of approximation by iterated multilayer neural network operators of the last four types. These approximations are achieved by establishing multidimensional Jackson type inequalities involving the multivariate modulus of continuity of the engaged function or its partial derivatives. Our multivariate operators are defined by using a multidimensional density function induced by a \(q\)-deformed and \(\lambda\)-parametrized hyperbolic tangent function, which is a sigmoid function. The approximations are pointwise and uniform. The related feed-forward neural network are with one or multi hidden layers.
Full text PDF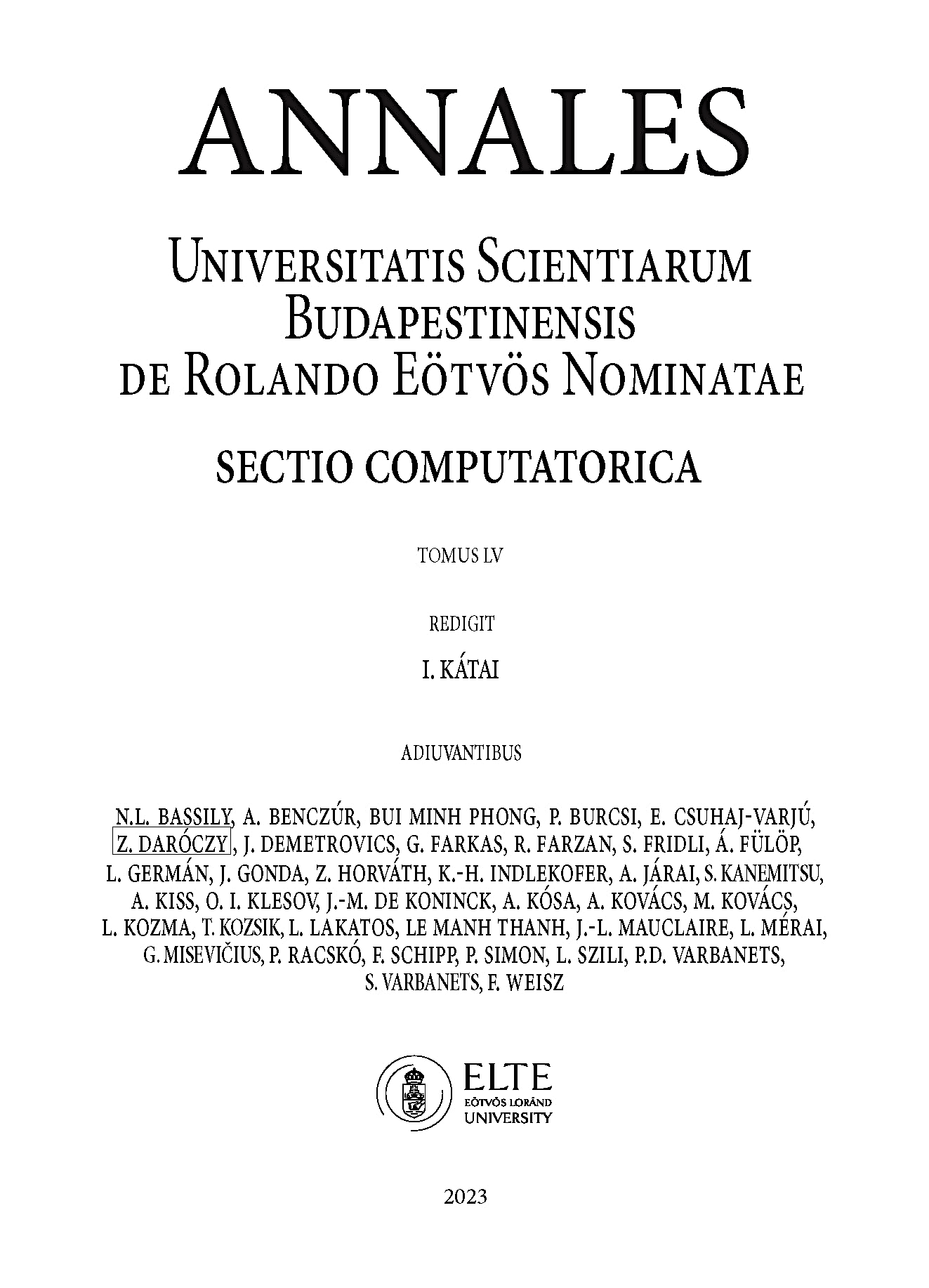